À propos du projet LANDSURF
Buts et objectifs
Le continent africain est confronté à divers défis et à de nombreux risques en raison du
changement et de la variabilité climatiques passés et présents. Pour renforcer la
résilience des sociétés ouest-africaines dans les secteurs de l'agriculture, de la
sécurité alimentaire, de l'eau et de la gestion des risques, des mesures d'adaptation
doivent être planifiées et mises en œuvre à temps. La planification et la mise en œuvre
de mesures d'adaptation au changement climatique nécessitent des informations
climatiques fiables et facilement accessibles à l'échelle locale et communautaire,
adaptées aux besoins des différentes parties prenantes.
Dans ce contexte, le ministère fédéral allemand de l'éducation et de la recherche (BMBF)
a financé un projet intitulé Land surface processes as a determinant of climate change
in Africa (LANDSURF), afin d'identifier et de calculer des indices pertinents pour les
parties prenantes africaines du secteur agricole. Le projet LANDSURF fait partie de la
deuxième phase du plan d'action de recherche (WRAP2.0) du West African Science Service
Center on Climate Change and Adapted Land-Use (WASCAL), qui vise à générer des services
climatiques et environnementaux axés sur la demande pour soutenir la prise de décision
par les décideurs politiques et les autres parties prenantes du secteur agricole. D'où
le développement du système d'aide à la décision (DSS) pour l'agriculture, l'eau et la
gestion des risques en Afrique de l'Ouest. Avant le développement du système d'aide à la
décision, des ateliers initiaux ont été organisés à l'intention des parties prenantes,
au cours desquels certains indices spécifiques à l'agriculture ont été présentés,
discutés et validés. La liste d'indicateurs qui en a résulté a été utilisée comme
variables cibles de la base de données du DSS. Les indicateurs sont liés à un large
éventail d'aspects, notamment la température, les précipitations, la saison des pluies,
la sécheresse et les indices agricoles. Sur cette base, nous avons comparé et
sélectionné des données d'observation et de modélisation adéquates pour calculer les
indices requis pour le passé (1981-2010) ainsi que pour trois périodes futures jusqu'à
la fin du siècle dans l'hypothèse de deux scénarios différents d'émissions de gaz à
effet de serre. Comme données de modèle, nous avons inclus des modèles avec des
résolutions spatiales grossières et fines pour assurer une résolution à l'échelle des
processus de décision régionaux et locaux. Ainsi, les données et les indicateurs du DSS
peuvent servir de base à une prise de décision saine et éclairée sur les mesures
d'adaptation à prendre pour faire face aux menaces du changement climatique. Pour mieux
comprendre et interpréter les résultats, il est vivement conseillé aux utilisateurs du
MAS et de ses données de lire la documentation qui suit.
Objectifs principaux:
- Rendre les données obtenues dans le cadre du projet LANDSURF librement et facilement accessibles
- Co-développement de la conception et du contenu avec les parties prenantes
- Transfert, diffusion et distribution des connaissances, des informations climatiques et des données issues de la recherche
- Permettre aux parties prenantes et aux petits exploitants d'intégrer les données et les connaissances acquises dans leur processus de prise de décision
- Renforcer la résilience des acteurs et des petits exploitants d'Afrique de l'Ouest face au changement climatique
Partenaires du projet
-
Germany
Nom Discipline Prof. Dr. Heiko Paeth Climatologie Dr. Katrin Ziegler Climatologie Dr. Daniel Abel Climatologie Dr. Michael Thiel Télédétection Dr. Insa Otte Télédétection -
University of Halle-Wittenberg
Germany
Name Discipline Prof. Dr. Christopher Conrad Télédétection, Géoécologie Lorenz König Géoinformatique Dr. Mike Teucher Télédétection, Géoécologie -
HEREON - GERICS - Climate Service Center Hamburg
Germany
Name Discipline Dr. Torsten Weber Dr. María Máñez Costa Tania Guillén Bolaños * Simon Muwafu * -
Burkina Faso
Name Discipline Dr. Jean-Bosco Benewindé Zoungrana and team -
United Nations University Accra
Ghana
Name Discipline Dr. Ferdinand Tornyie and team -
Federal University of Technology Akure
Nigeria
Name Discipline Prof. Dr. Vincent Ajayi Météorologie et science du climat Dr. Imoleayo Ezekiel Gbode Météorologie et science du climat -
Niger
Name Discipline Dr. Seydou B. Traore Agroclimatologie Dr. Moussa Waongo Agroclimatologie Dr. Amadou Coulibaly Agroclimatologie -
* Former members
Le portail web - DSS
Qu'est-ce qu'un système d'aide à la décision (DSS)?
- Il s'agit d'un système informatique interactif et flexible
- Les DSS sont utilisés pour l'identification et la résolution de problèmes complexes
- Fournit des informations permettant de prendre la meilleure décision possible
Note
L'aide à la décision n'est ni une proposition de décision ni une décision elle-même. Ce portail vise à fournir des informations solides et efficaces de manière exhaustive.
Évaluation du DSS
Une partie importante du processus de co-conception et de co-développement est l'évaluation du DSS par les parties prenantes et les utilisateurs. À cette fin, le projet LANDSURF a organisé un atelier virtuel sur l'évaluation d'une version bêta du système en juin 2023. Lors de cet atelier, jusqu'à 28 participants de différents pays et institutions ont participé à l'atelier virtuel et ont fourni un retour d'information sur la première application du DSS. Pour ce faire, l'accès au système a été donné quelques semaines à l'avance afin de permettre aux parties prenantes de tester le système et sa convivialité. Au cours de l'atelier, le DSS a été présenté au public et des exemples d'application ont été donnés par les développeurs. En outre, les réactions et les contributions supplémentaires des utilisateurs ont été discutées et résumées dans une enquête complémentaire. Les résultats sont rassemblés dans le résumé (english summary, french summary). Les développeurs ont également fait part aux parties prenantes des informations supplémentaires qu'il était prévu d'inclure. Quelques semaines après l'atelier, les parties prenantes et les utilisateurs ont été invités à donner leur avis sur le DSS dans le cadre d'une enquête plus détaillée. Tous les commentaires reçus ont permis de développer le contenu et la convivialité du DSS.
Décisions spatiales - SDSS
Notre système peut être décrit comme un système d'aide à la décision spatiale (SDSS)
- Les cartes jouent un rôle décisif dans les processus d'aide à la décision
- L'utilisation de cartes permet de réduire le temps de décision
- Améliore la compréhensibilité et la précision des résultats
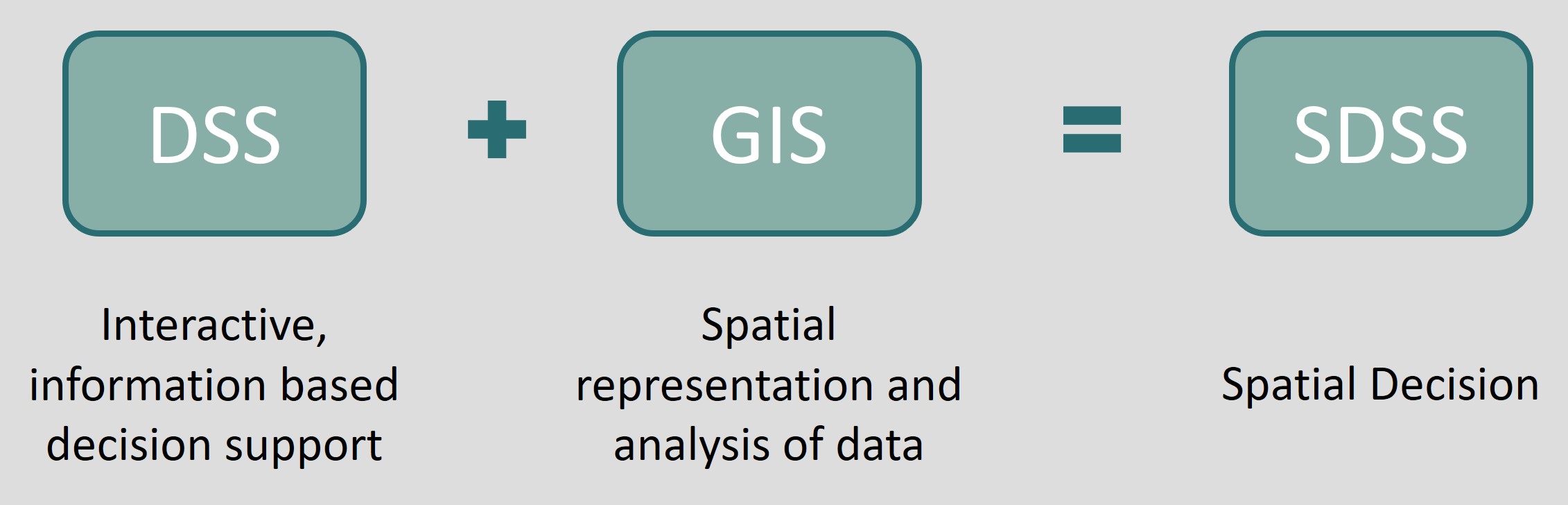
fig. 1: Schéma d'un SDSS