About the LANDSURF Project
Goals and Objectives
The African continent faces various challenges and numerous risks due to past and current
climate change and variability. To strengthen the resilience of West African societies
in the sectors of agriculture, food security, water and risk management, adaptation
measures need to be planned and implemented in time. Planning and implementing climate
change adaptation measures requires reliable and easily accessible local to
community-scale climate information tailored to the needs of different stakeholders.
Against this background, the German Federal Ministry of Education and Research (BMBF)
funded a project, Land surface processes as a determinant of climate change in Africa
(LANDSURF), to identify and calculate indices relevant to African stakeholders in the
agricultural sector. The LANDSURF project is part of the second phase of the West
African Science Service Center on Climate Change and Adapted Land-Use (WASCAL) Research
Action Plan (WRAP2.0), which aims to generate demand-driven climate and environmental
services to support decision making by policy makers and other stakeholders in the
agricultural sector. Hence the development of the Decision Support System (DSS) for
Agriculture, Water and Risk Management in West Africa. Prior to the development of the
DSS, initial stakeholders’ workshops were held, where some relevant agriculture-specific
indices were presented, discussed and validated. The resulting list of indicators was
used as the target variables of the DSS database. The indicators are related to a wide
range of aspects, including temperature, precipitation, the rainy season, drought, and
agricultural indices. On this basis, we compared and selected adequate observation and
model data to calculate the required indices for the past (1981-2010) as well as for
three future periods until the end of the century under the assumption of two different
greenhouse gas emission scenarios. As model data, we included models with coarse and
fine spatial resolutions to ensure a resolution being on the scale of regional to local
decision processes. Thus, the data and indicators in the DSS are able to set a ground
for sound and informed decision-making on adaptation measures to face the threats of
climate change. To better understand and interpret the results, users of the DSS and its
data are strongly advised to read the subsequent documentation.
Main Goals:
- Make obtained data from the LANDSURF project freely and easily accessible
- Co-development of design and contents together with stakeholders
- Transfer, dissemination and distribution of knowledge, climate information and data from research
- Enable stakeholders and smallholders to incorporate the data and insights gained into their decision-making process
- Strengthen resilience of West African stakeholders and smallholders in regard to climate change
Project Partners
-
Germany
Name Institute Prof. Dr. Heiko Paeth Climatology Dr. Katrin Ziegler Climatology Dr. Daniel Abel Climatology Dr. Michael Thiel Remote Sensing Dr. Insa Otte Remote Sensing -
University of Halle-Wittenberg
Germany
Name Discipline Prof. Dr. Christopher Conrad Remote Sensing, Geoecology Lorenz König Geoinformatics Dr. Mike Teucher Remote Sensing, Geoecology -
HEREON - GERICS - Climate Service Center Hamburg
Germany
Name Discipline Dr. Torsten Weber Dr. María Máñez Costa Tania Guillén Bolaños * Simon Muwafu * -
Burkina Faso
Name Discipline Dr. Jean-Bosco Benewindé Zoungrana and team -
United Nations University Accra
Ghana
Name Discipline Dr. Ferdinand Tornyie and team -
Federal University of Technology Akure
Nigeria
Name Discipline Prof. Dr. Vincent Ajayi Meteorology and Climate Science Dr. Imoleayo Ezekiel Gbode Meteorology and Climate Science -
Niger
Name Discipline Dr. Seydou B. Traore Agroclimatology Dr. Moussa Waongo Agroclimatology Dr. Amadou Coulibaly Agroclimatology -
* Former members
The Web Portal - DSS
What is a Decision Support System (DSS)?
- It's an interactive and flexible computer based system
- DSS's are used for the identification and solution of complex problems
- Provides information to support making the best possible decision
Note
Decision support is neither a decision proposal nor a decision itself. This portal aims to give solid and efficient information in a comprehensive way.
Evaluation of the DSS
An important part of the co-design and co-development process is the evaluation of the DSS by the stakeholders and users. To this end, the LANDSURF project conducted a virtual workshop on the evaluation of a beta version of the system in June 2023. In this workshop, up to 28 participants from various countries and institutions participated in the virtual workshop and provided feedback on the first application of the DSS. This was achieved by providing access to the system a few weeks in advance to give the stakeholders the opportunity to test the system and its user friendliness. During the workshop, the DSS was presented to the audience and application examples were given from the developers’ side. Further, feedback and additional input from the users were discussed and summarised in a complementing survey. The results are collected in the summary (english summary, french summary). The developers also provided some input to the stakeholders regarding further information planned to be included. A few weeks after the workshop, stakeholders and users were asked for their views on the DSS in a more detailed survey. All input we received helped to further develop the content and user friendliness of the DSS.
Spatial Decisions - SDSS
Our system can be described as a spatial decision support system (SDSS)
- Maps play a decisive role in decision support processes
- Usage of maps reduce decision time
- Increases understandability and accuracy of the results
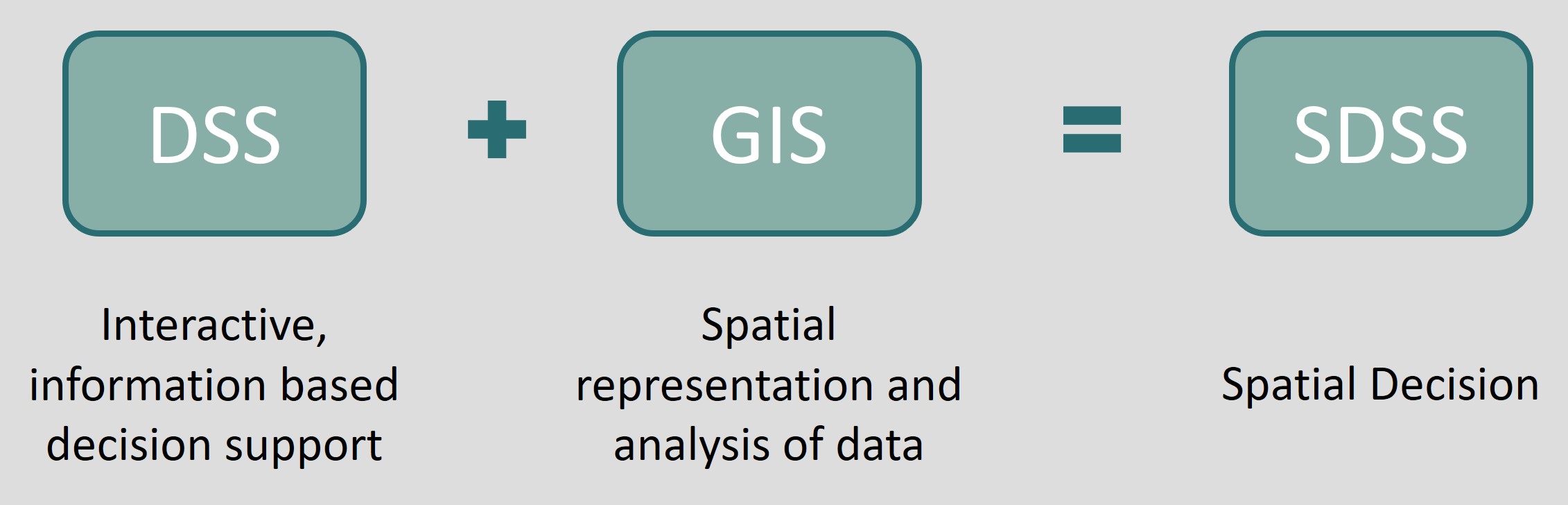
fig. 1: Scheme of a SDSS